Predictive Synthesis
--- Predictive synthesis of ceramic materials
With the aid of high-throughput computation, we can generate hundreds or thousands of materials in a relatively short time. However, making them happen in experiments usually take a much longer time. This is particularly complicate for inorganic ceramic materials given that there could be many synthesis options while on the other hand a lot of side reactions may happen. In this part of research, I am interested in developing predictive theory to provide experimental synthesis instructions. It covers typical synthesis conditions such as temperature, composition, pH etc., as well as unconventional synthesis conditions, such as curvature, external strain, electrostatic gating, eletric field etc. I hope my research can not only provide tips for rationale the experimentation design, but also contibute to the realization of autonomous synthesis development for ceramic materials.
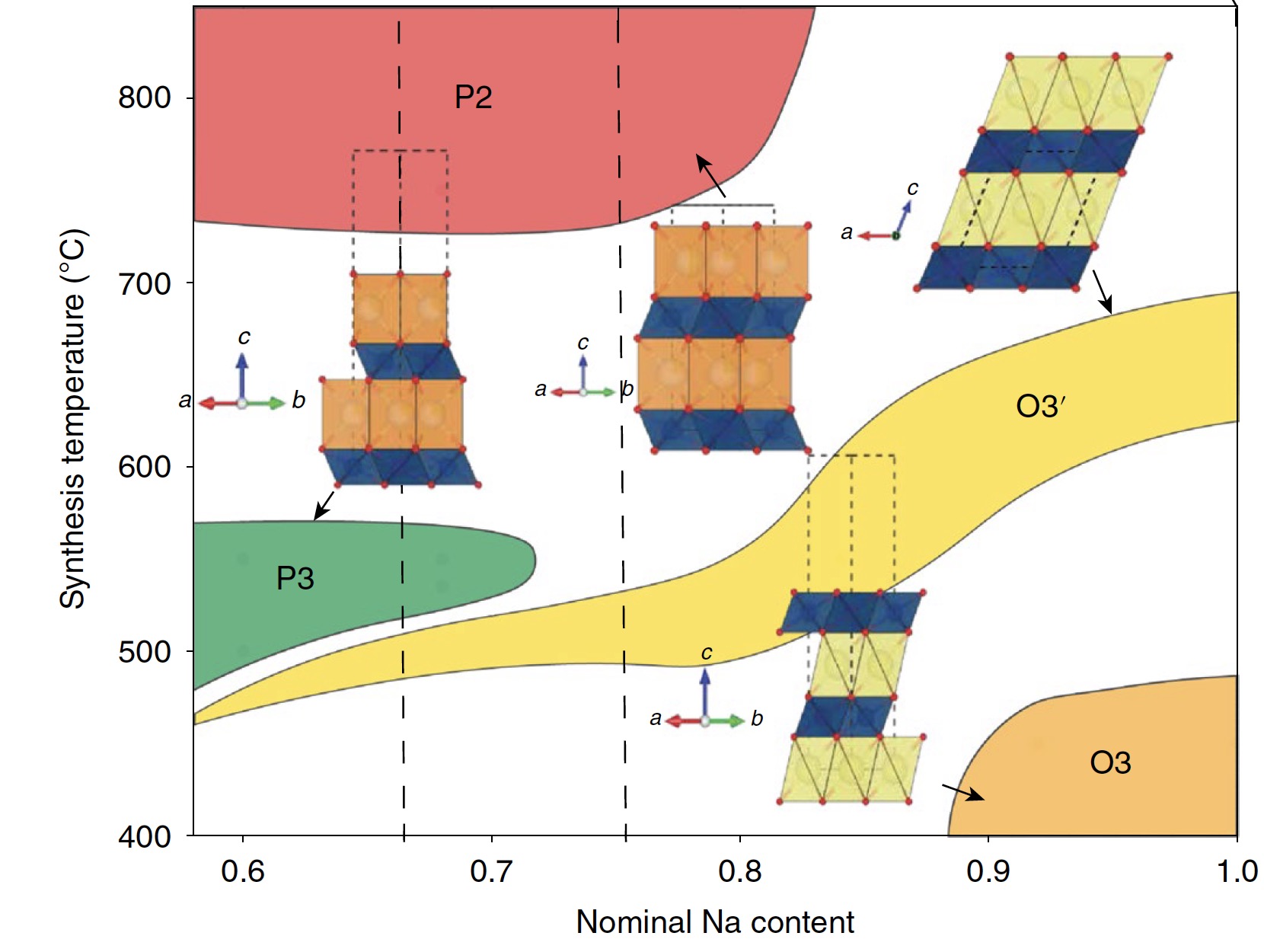
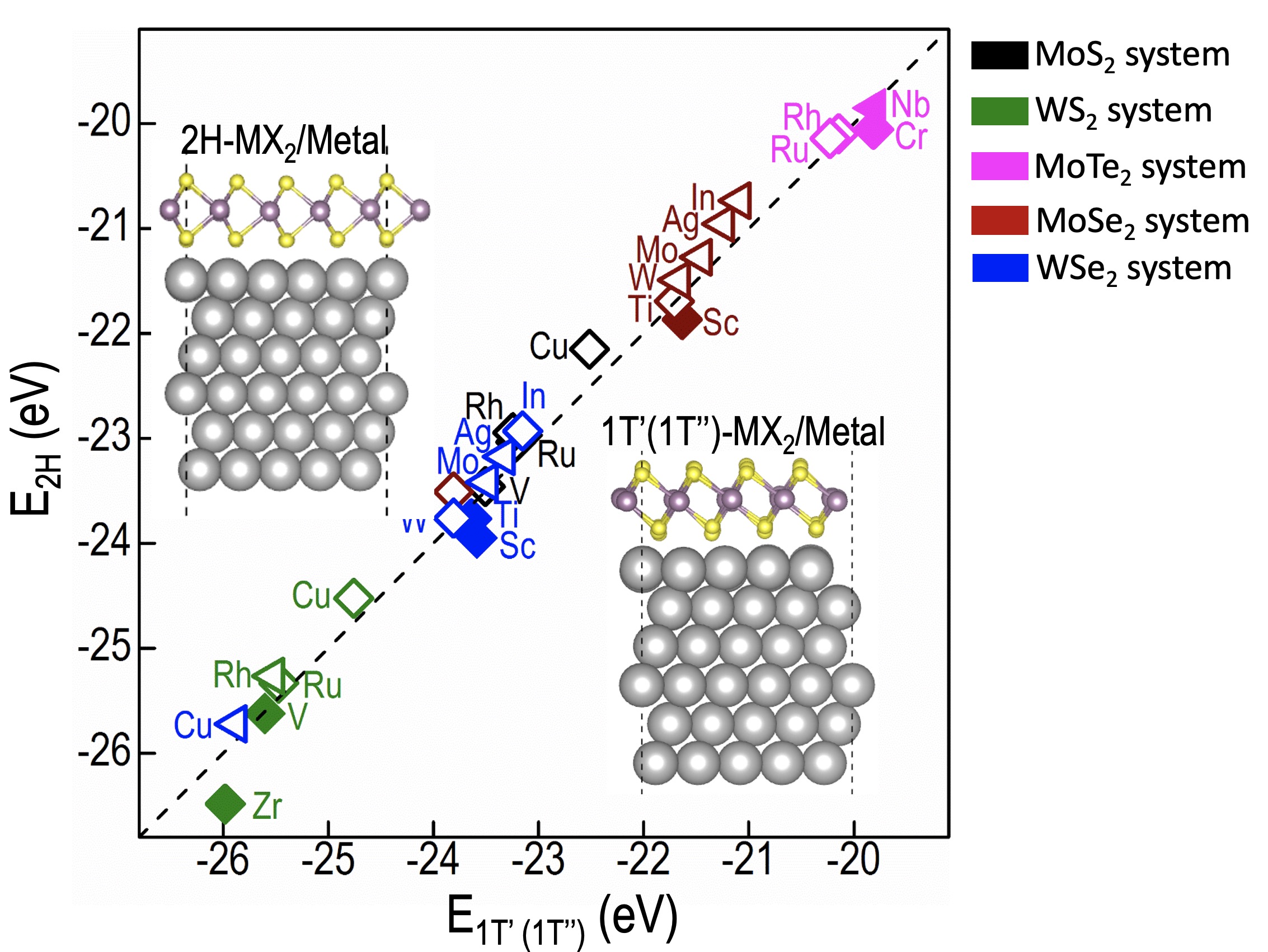
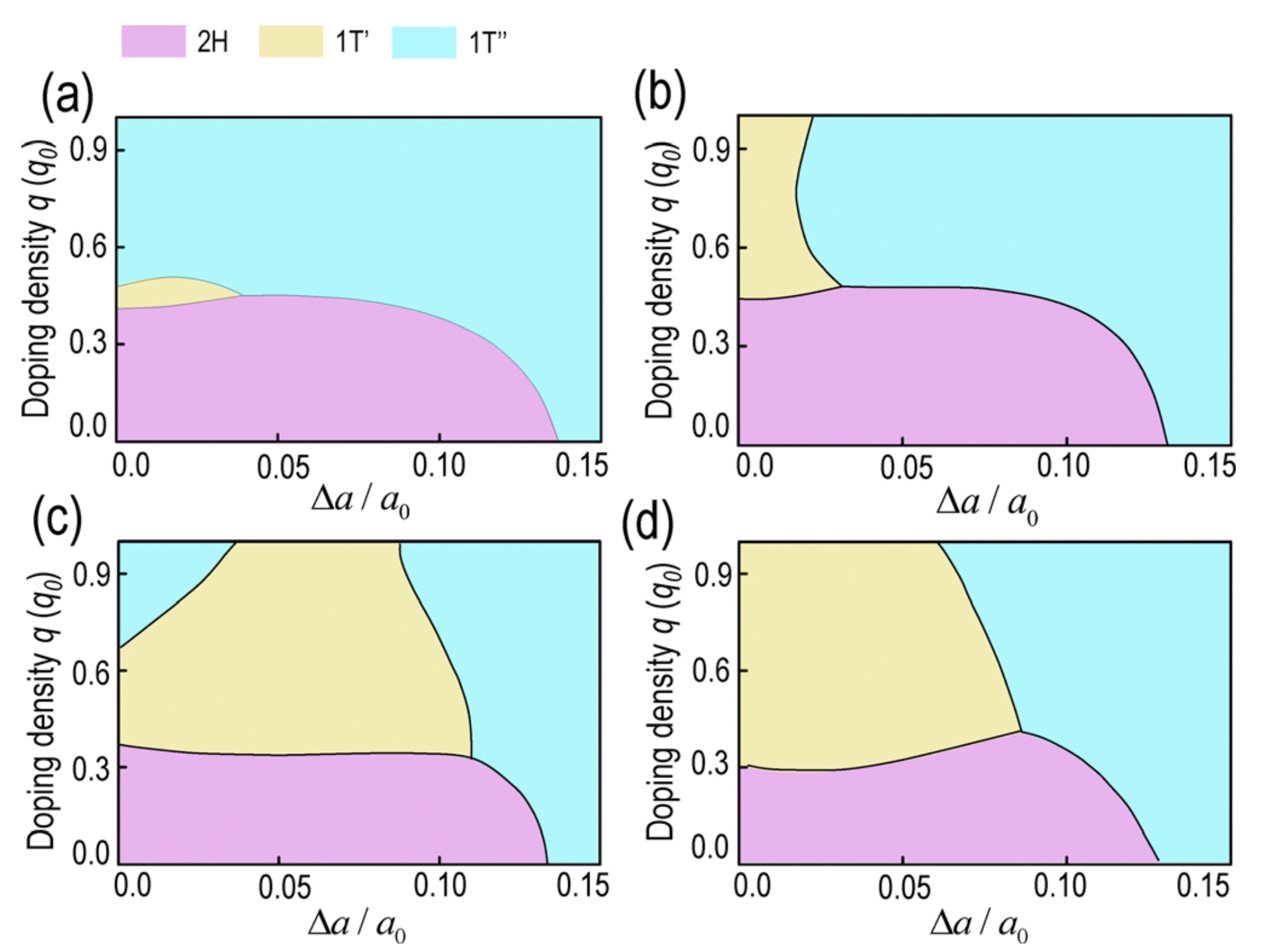
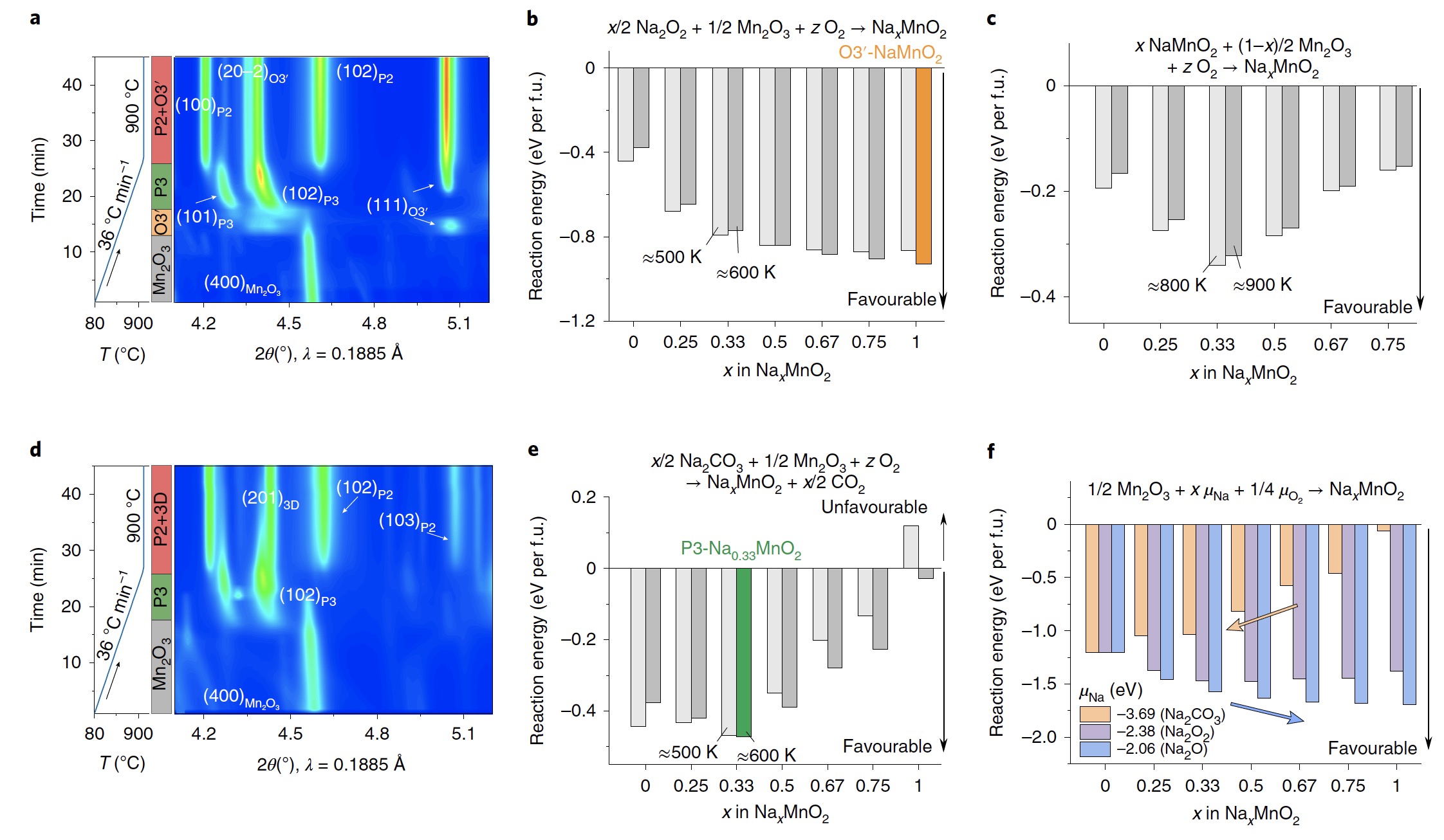